Improve Workflows & Cost Savings
AI-powered space optimization leverages machine learning algorithms to analyze storage patterns, improving warehouse capacity and operational efficiency while reducing costs and enhancing supply chain reliability.
The Problem
Order picking is the most resource-intensive process and it highly depends on storage location policy. For instance, regarding storage layouts, many warehouses underutilize vertical space, leading to wasted capacity and increased storage costs. This can be due to outdated storage systems, lack of proper racking infrastructure, or inefficient stacking practices. Additionally, static layouts, blind to shifting inventory needs and demand, force pickers to travel needlessly – a staggering 55% of their time. This drags down efficiency and creates bottlenecks that delay orders.
The AI Opportunity
AI-driven solutions optimize warehouse space through dynamic product clustering, calculating ideal product placements to streamline picking routes. This reduces unnecessary travel within warehouses, improving space utilization, operational efficiency, and cost savings. With AI, warehouses can dynamically adapt to inventory changes and demand patterns, ensuring streamlined workflows and scalable efficiency gains.
Why It Matters
Optimizing warehouse space improves operational efficiency, reduces costs, and enhances customer satisfaction. Businesses can better meet market demands while reducing their environmental footprint by minimizing unnecessary expansions. With smarter space management, organizations gain a competitive edge, ensuring long-term scalability and sustainability.
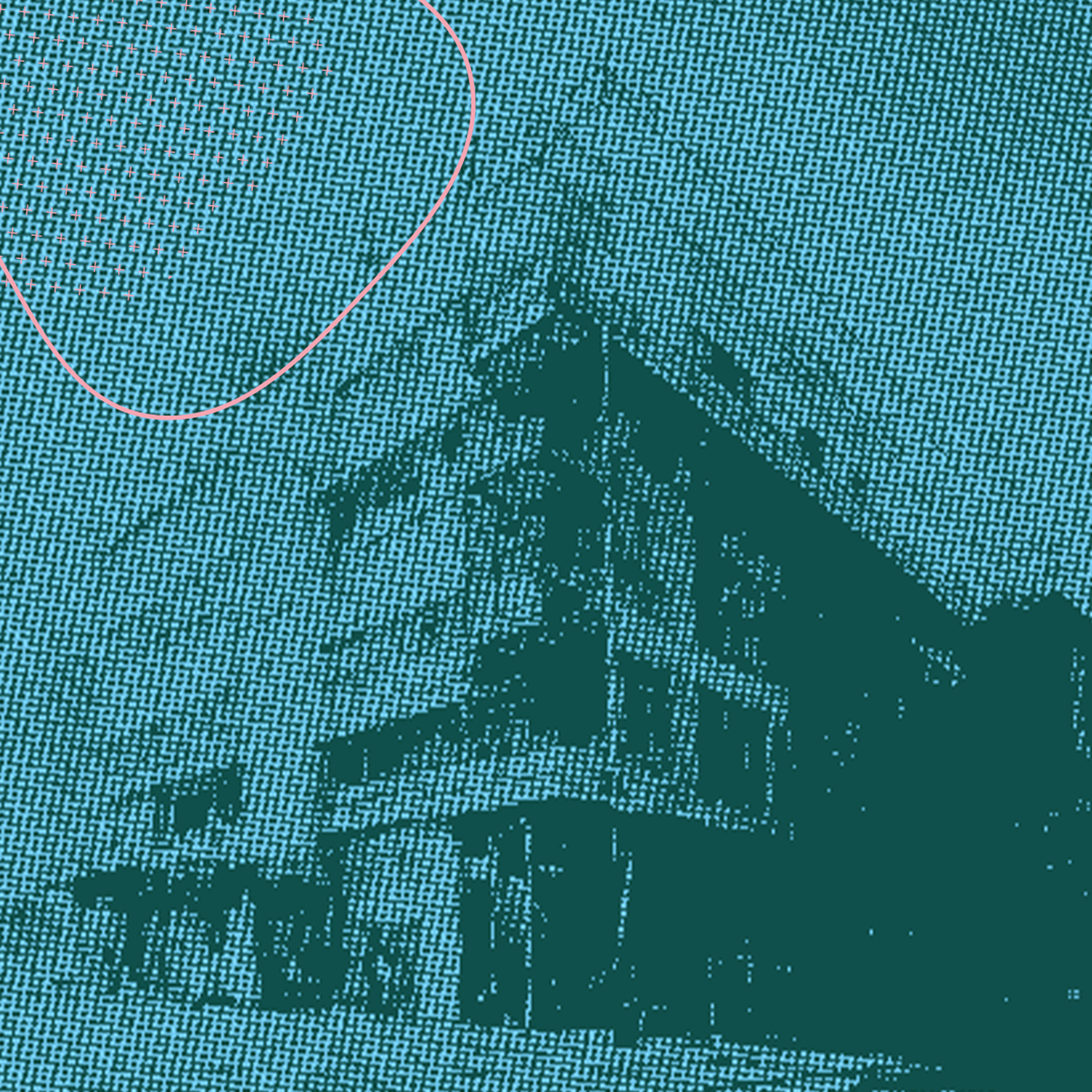
Benefits & Impact
Cost Savings
Optimized storage layouts lower operational expenses by reducing unnecessary labor costs and streamlining picking processes, enabling warehouses to handle more inventory with fewer resources.
Improved Operational Efficiency
Better organization reduces order-picking time, minimizing errors and enhancing customer satisfaction.
Capacity Gains
Inefficient use of storage space limits warehouse capacity and increases costs, which ML can address dynamically.
AI Methods & Models

Build Your AI Solution with Amii
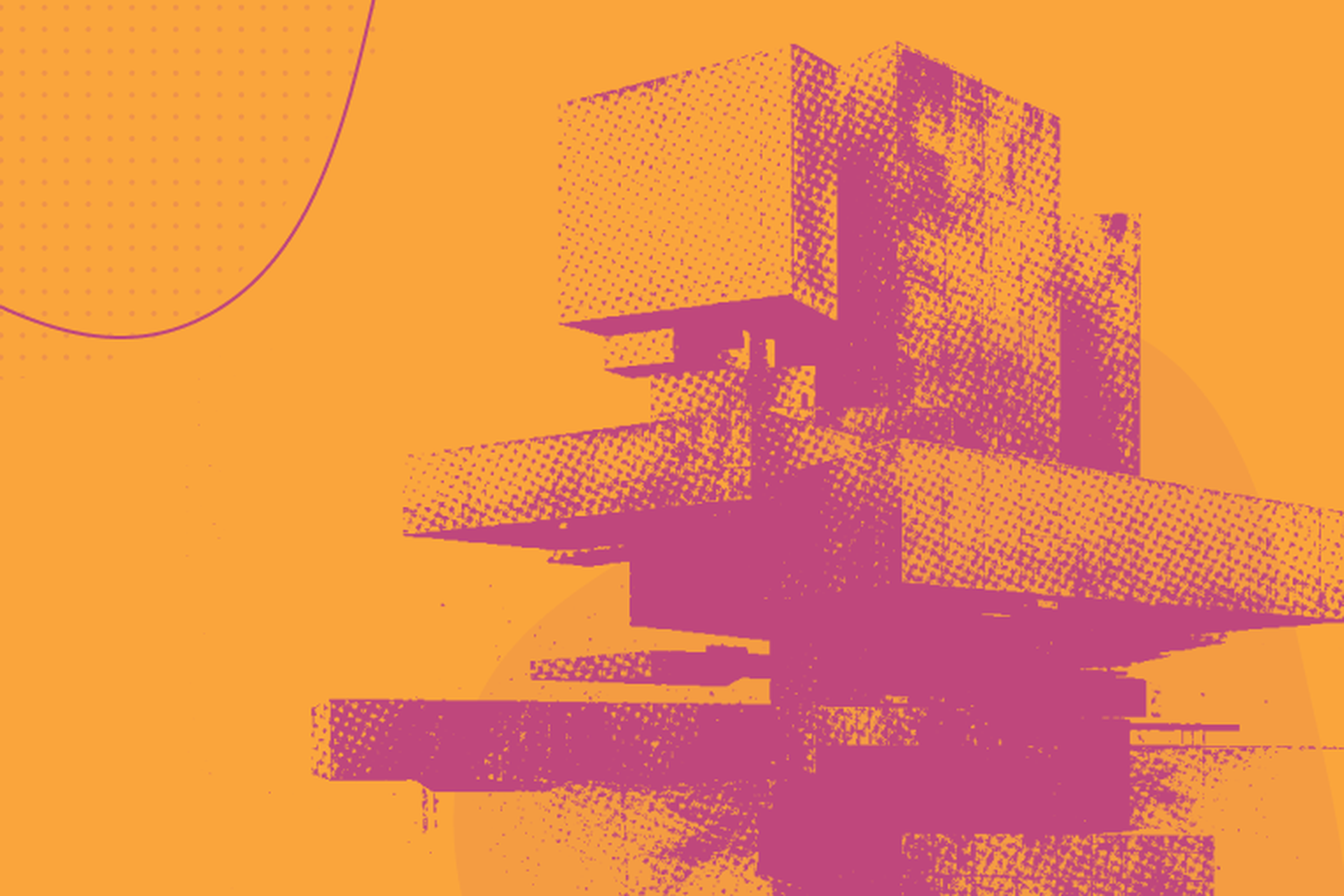
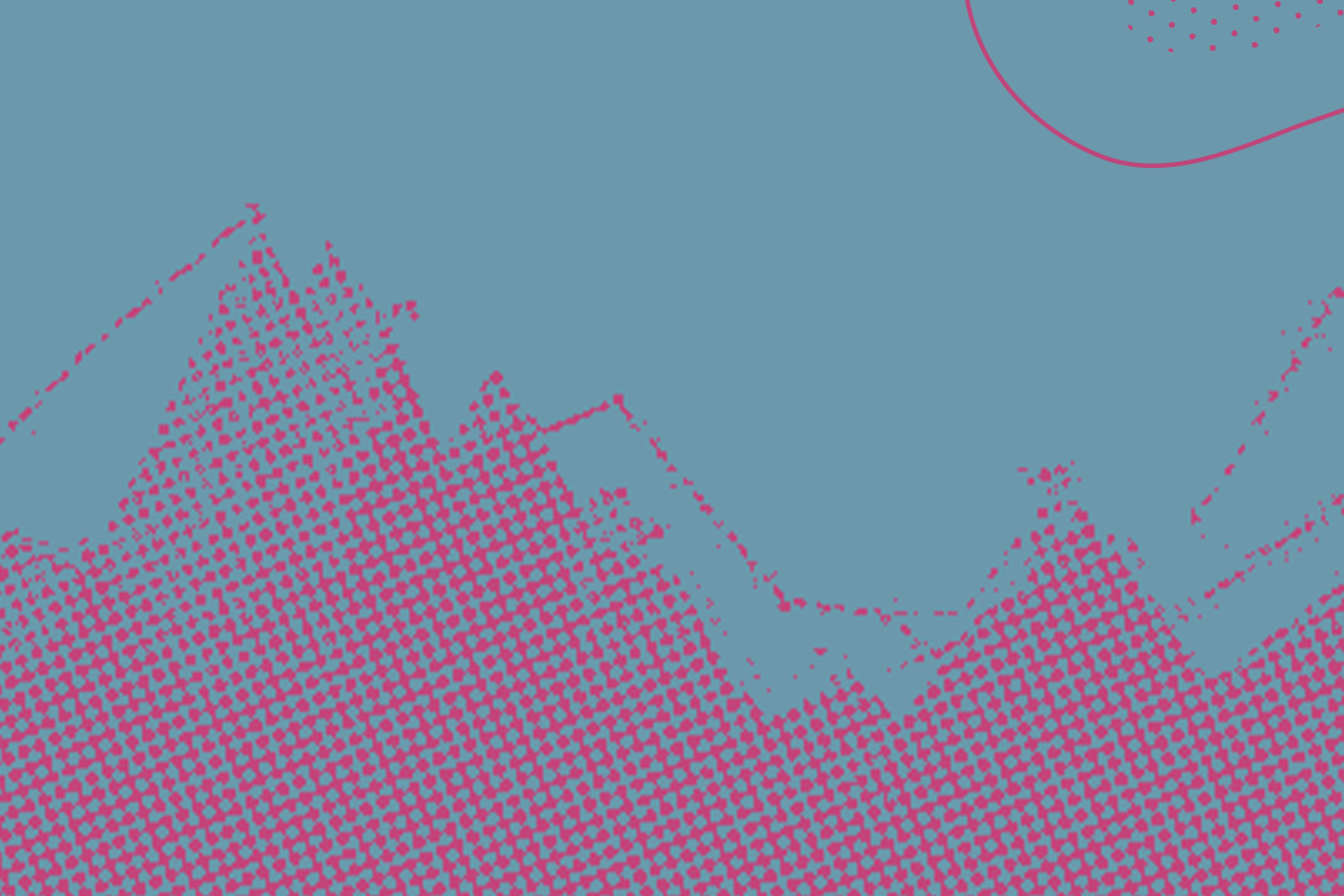
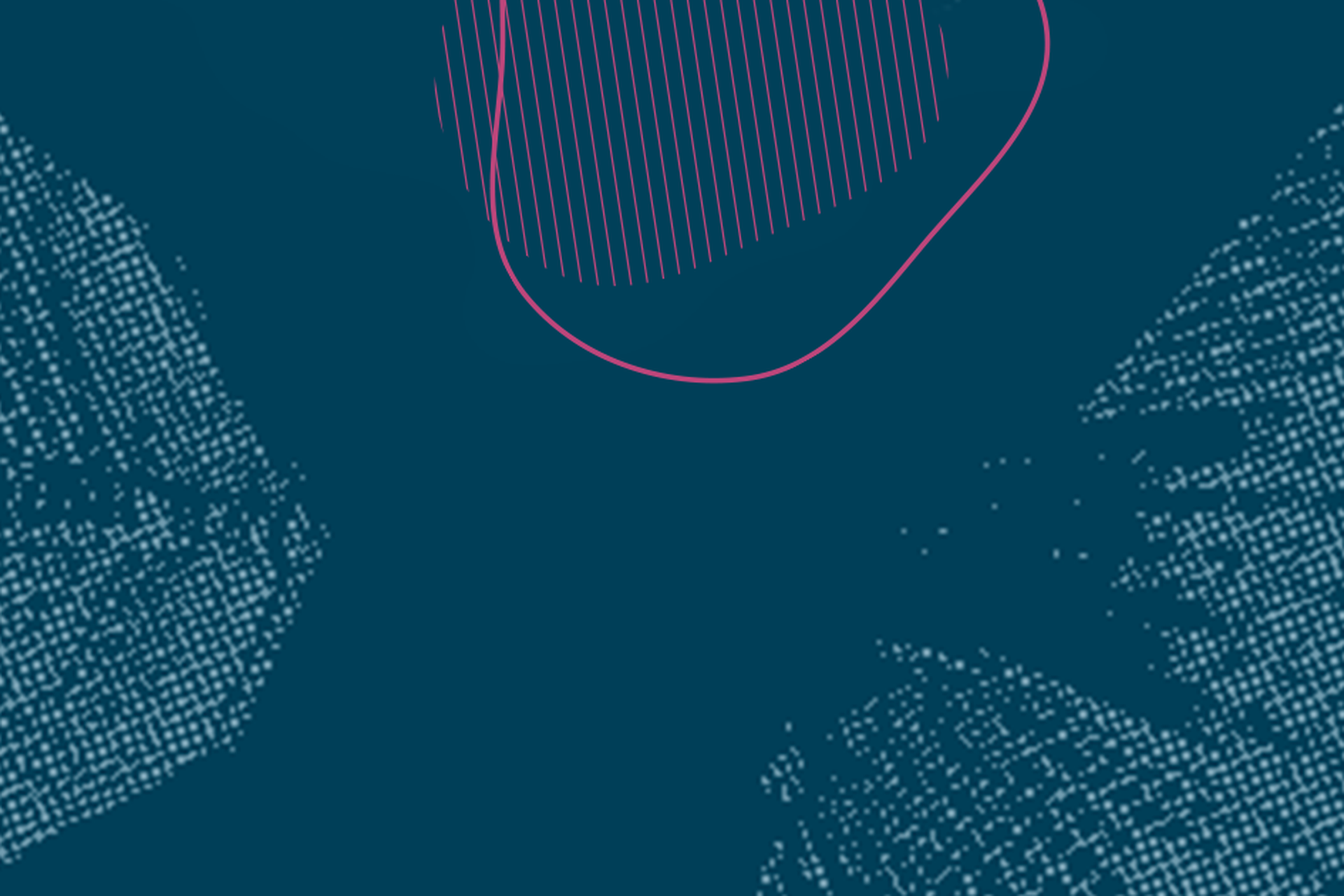
Development
Sources
Flognman, E., Grönlund, E., & Ticehurst Falk, M. (2021). Optimizing Warehouse Logistics with Artificial Intelligence : Market analysis (Dissertation).
Janse van Rensburg, L. (2019). Artificial Intelligence for Warehouse Picking Optimization - An NP-Hard Problem (Dissertation).
Rodrigo Furlan de Assis, Alexandre Frias Faria, Vincent Thomasset-Laperrière, Luis Antonio Santa-Eulalia, Mustapha Ouhimmou, William de Paula Ferreira. Machine Learning in Warehouse Management: A Survey. Procedia Computer Science. Volume 232, 2024, Pages 2790-2799.