Improve R&D Sustainability
AI-driven predictive analytics optimizes drug development by reducing inefficiencies, improving success rates, and cutting costs. These advancements lead to faster market entry, higher ROI, and a more sustainable R&D pipeline.
The Problem
Drug development faces significant inefficiencies, with 90% of candidates failing due to inefficacy or toxicity at the clinical trial phase. This results in substantial financial losses, delays in patient treatments, and underutilized R&D investments, limiting companies' profitability and sustainability.
The AI Opportunity
AI leverages historical data to predict drug efficacy and toxicity early, helping companies avoid late-stage failures. This ensures efficient allocation of resources, reduces trial durations, and improves the likelihood of successful market entry.
Organizations using AI-driven approaches have seen success in getting through preclinical stages quicker and cheaper than traditional methods, with some reporting savings of up to 30% in time and cost.
Why It Matters
AI reduces the financial burden of failed trials and accelerates timelines, enabling pharmaceutical companies to optimize their R&D budgets. Beyond profits, this creates a sustainable pipeline by minimizing resource use and improving access to life-saving treatments.
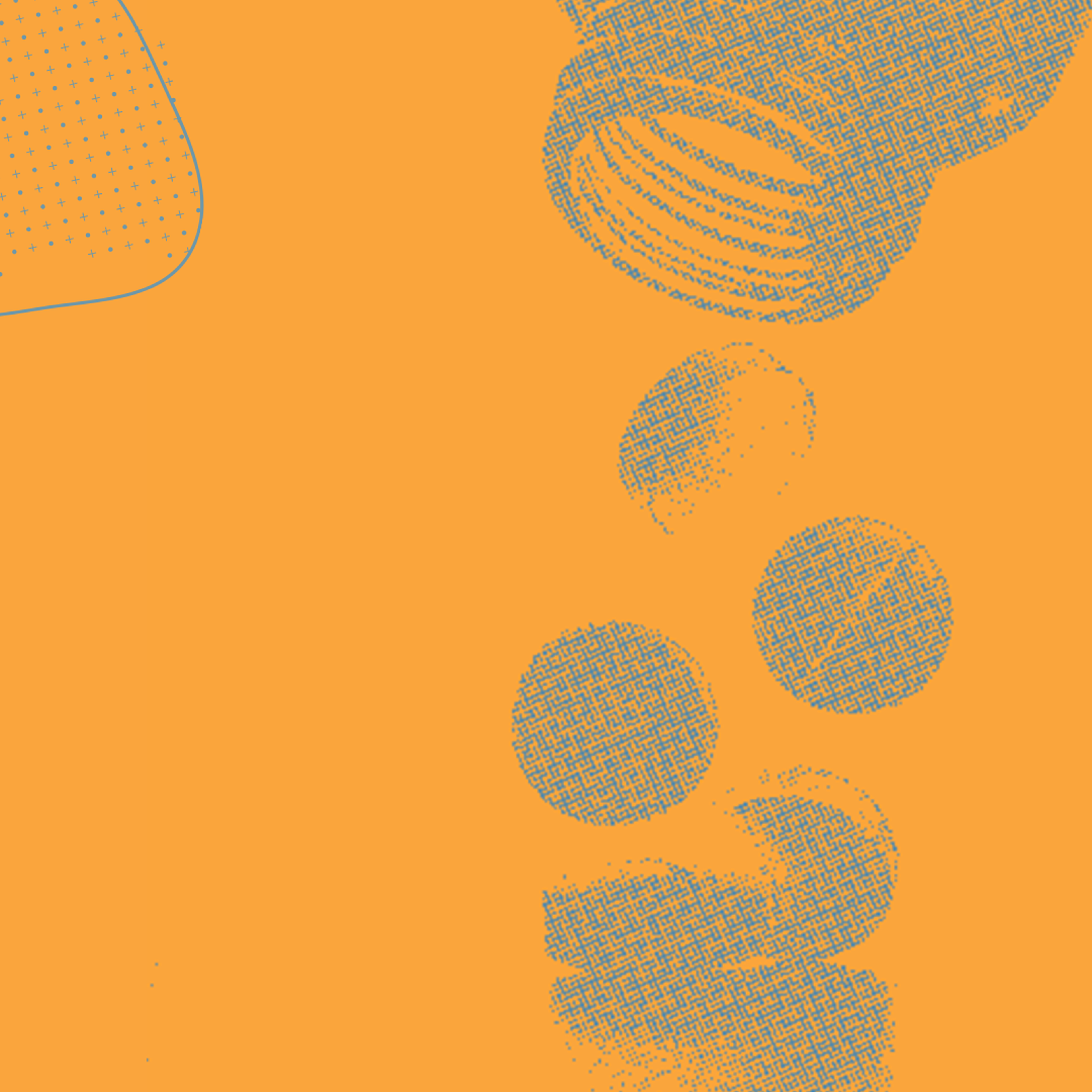
Benefits & Impact
Cost Reduction
AI streamlines the drug development process by identifying high-potential candidates early, minimizing resource expenditure on unsuccessful trials.
Revenue Acceleration
Faster and more efficient trials enable companies to bring new drugs to market sooner, maximizing the period of exclusivity and boosting profitability.
Sustainability Gains
By reducing waste in failed trials and optimizing resource use, AI supports environmentally and operationally sustainable practices in drug R&D, aligning with long-term corporate responsibility goals.
AI Methods & Models
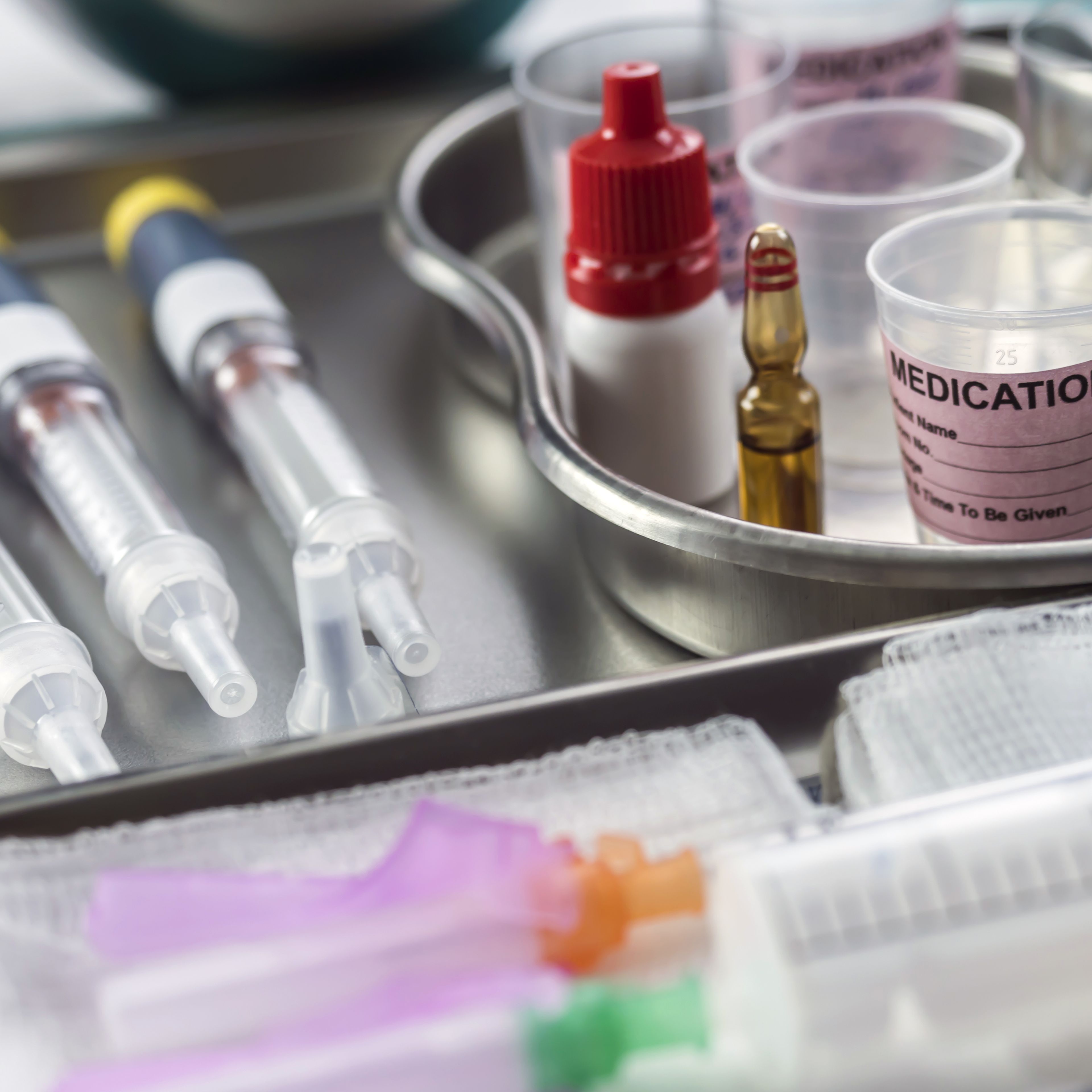
Build Your AI Solution with Amii
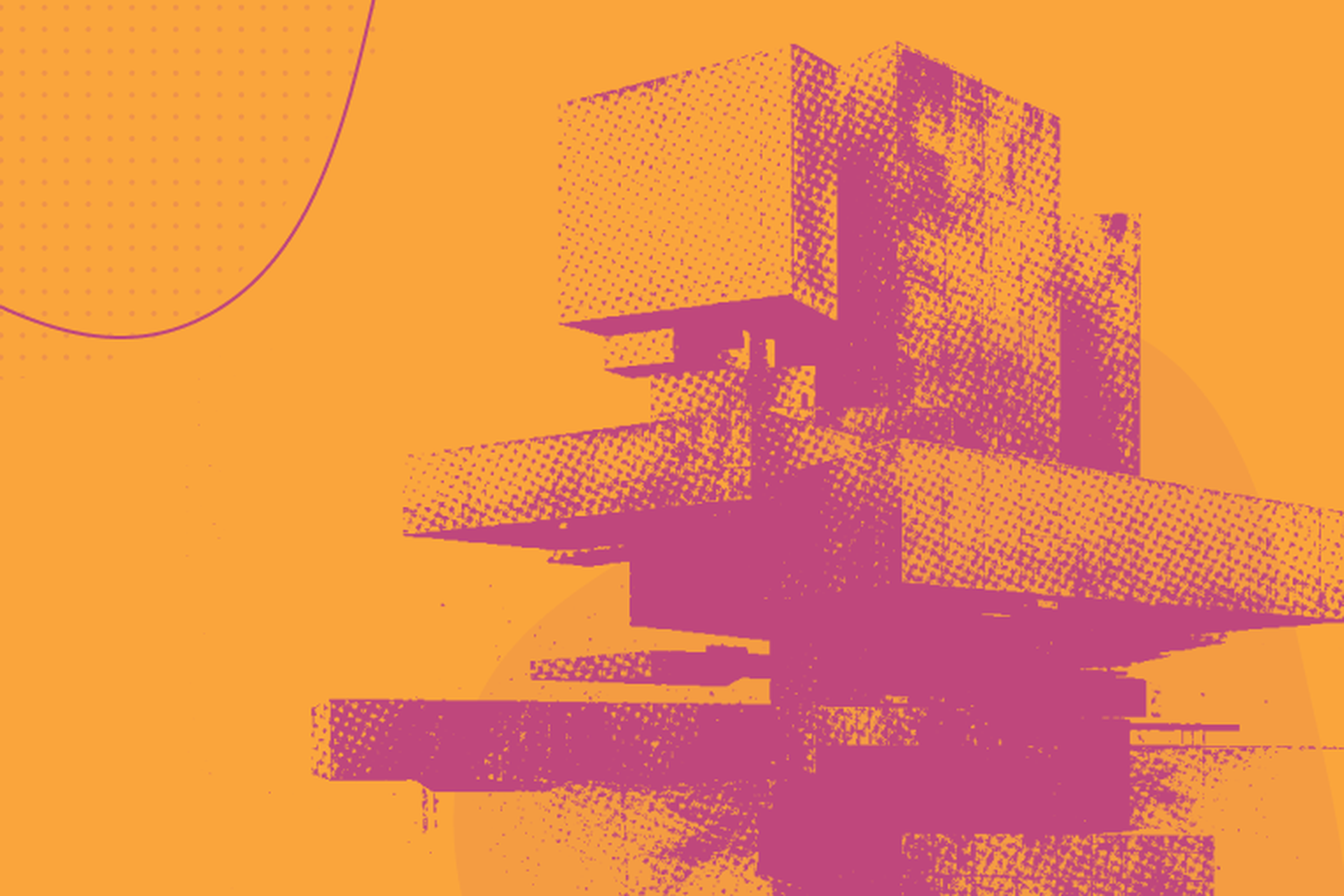
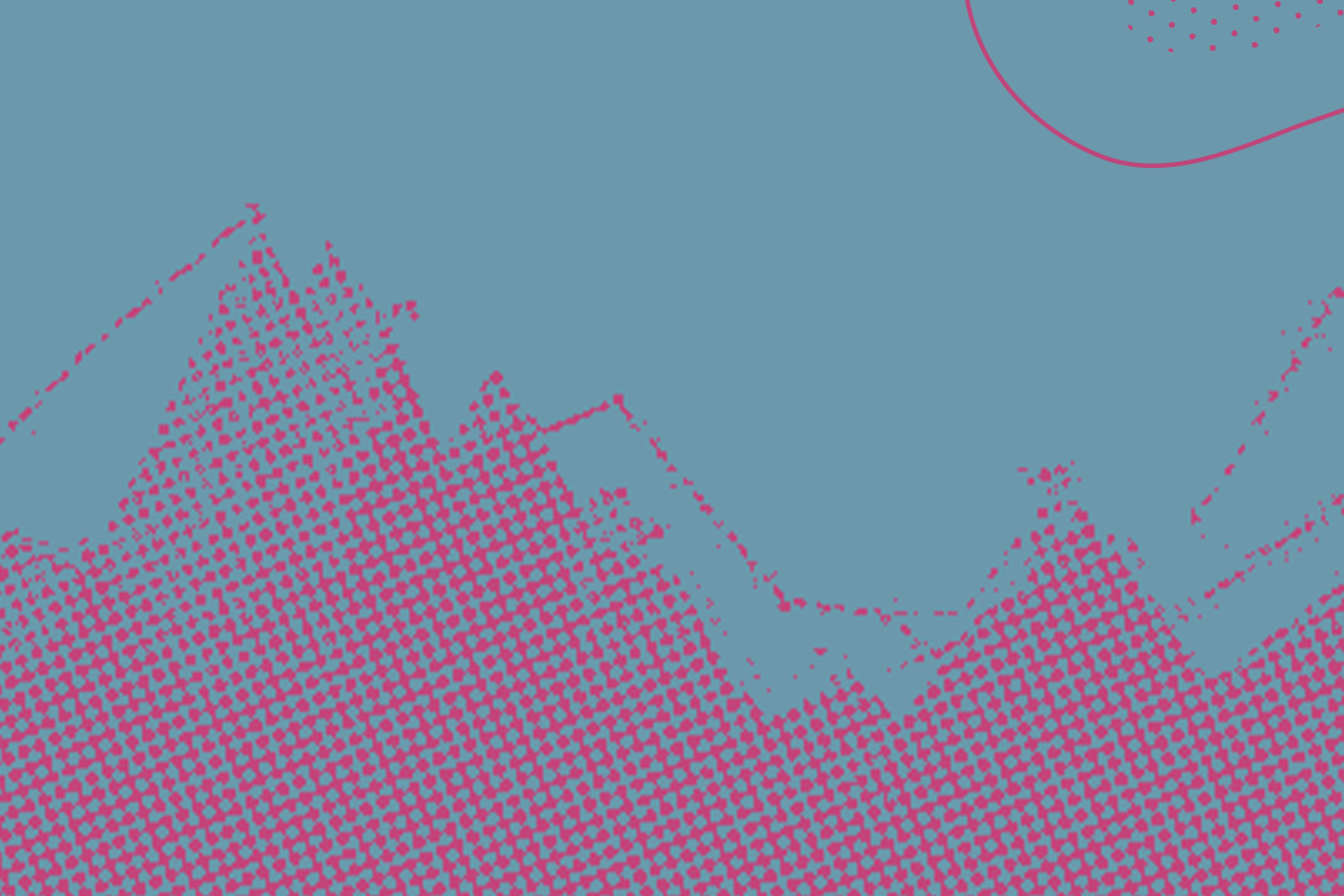
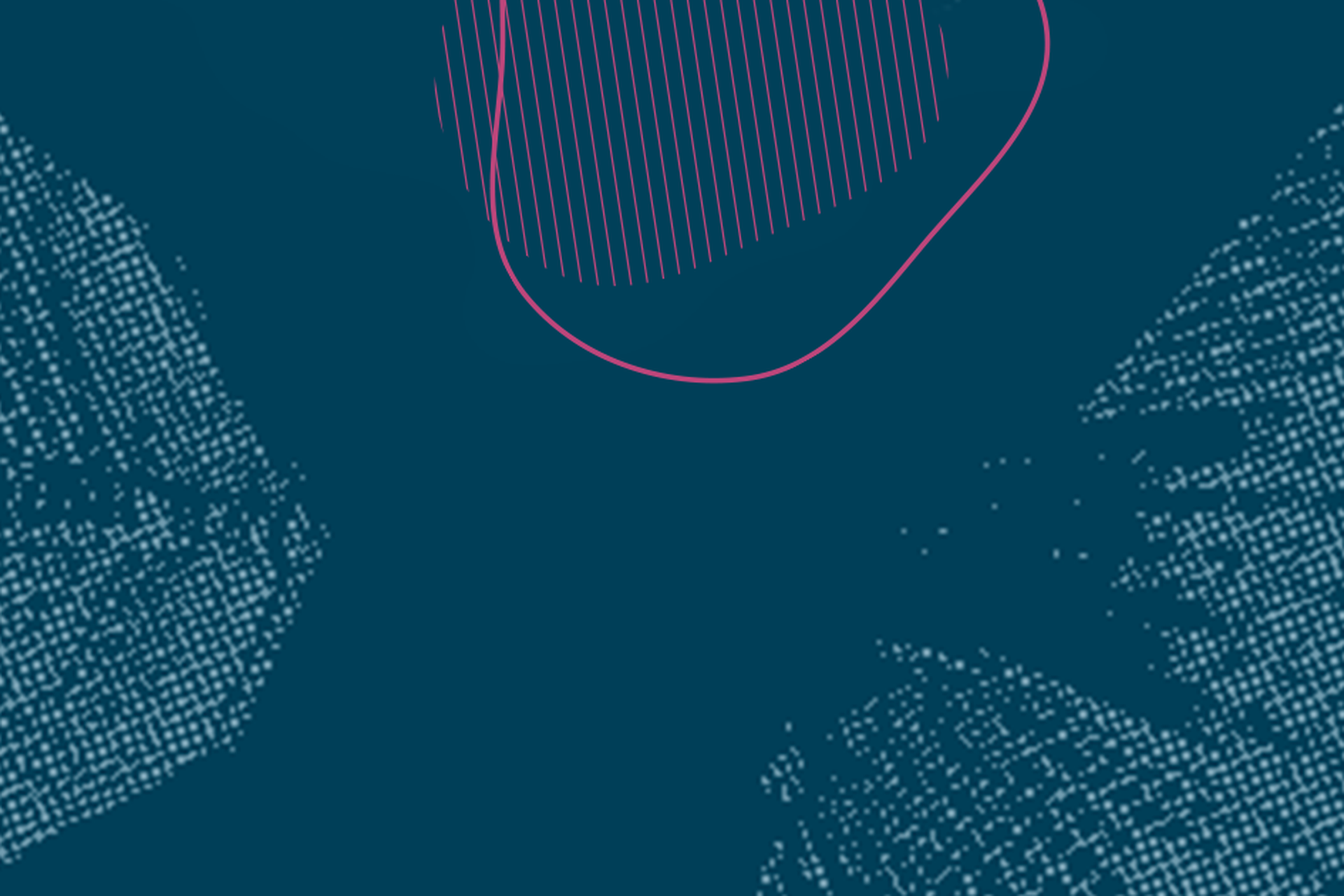
Development
Sources
Sun, D., Gao, W., Hu, H., & Zhou, S. (2022). Why 90% of clinical drug development fails and how to improve it? Acta Pharmaceutica Sinica B, 12(7), 3049–3062
Wellcome: Unlocking the Potential of AI in Drug Discovery (2023)