Loading...
Advancements in large language models are happening at a dizzying rate, with the seemingly constant news of bigger and more complex models.
But that comes at a cost. But bigger isn’t the only path forward. As models increase in complexity,, they also become more expensive, time-consuming to train, and resource-hungry. That's why there’s growing excitement around alternatives like Deepseek's R1—a model that delivers impressive results with a fraction of the cost and resources of comparable LLMs. This signals a shift in AI development where efficiency, not just scale, is driving innovation.
On the latest episode of the Approximately Correct podcast, Lili Mou — Amii Fellow and Canada CIFAR AI Chair — talks about his research into improving LLM efficiency and how smaller, more accessible models could expand opportunities for everyone.
"I think there are two general directions,” he tells hosts Alona Fyshe and Scott Lilwall.“One is bigger and bigger. But the other is smaller and smaller — and it can change our life in all kinds of setups.”
Research into LLM efficiency is reshaping what's possible, making smaller, more accessible models a reality.
This could shift could suddenly make custom LLMs development more accessible for smaller organizations.
“For startup companies, they don't have the budget for building the big clusters or they don't have data and can’t collect tons of samples to train the models,” he says.
It would also be a game-changer for industries like vital for industries that deal with sensitive information and privacy concerns, like healthcare or financial services, where data needs to be more tightly controlled.
“So the question is, how can I make my language model more efficient?
Mou explains how techniques like low-rank projection and multi-teacher distillation are making LLMs smaller, faster, and more efficient. His contributions include developing Flora, an approach that allows LLMs to show superior performance while taking far less computing power to train.
Listen or watch the full interview to learn more about Mou’s work and what it could mean for the future of LLMs.
Approximately Correct: An AI Podcast from Amii is hosted by Alona Fyshe and Scott Lilwall. It is produced by Lynda Vang, with video production by Chris Onciul. Subscribe to the podcast on Apple Podcasts or Spotify.
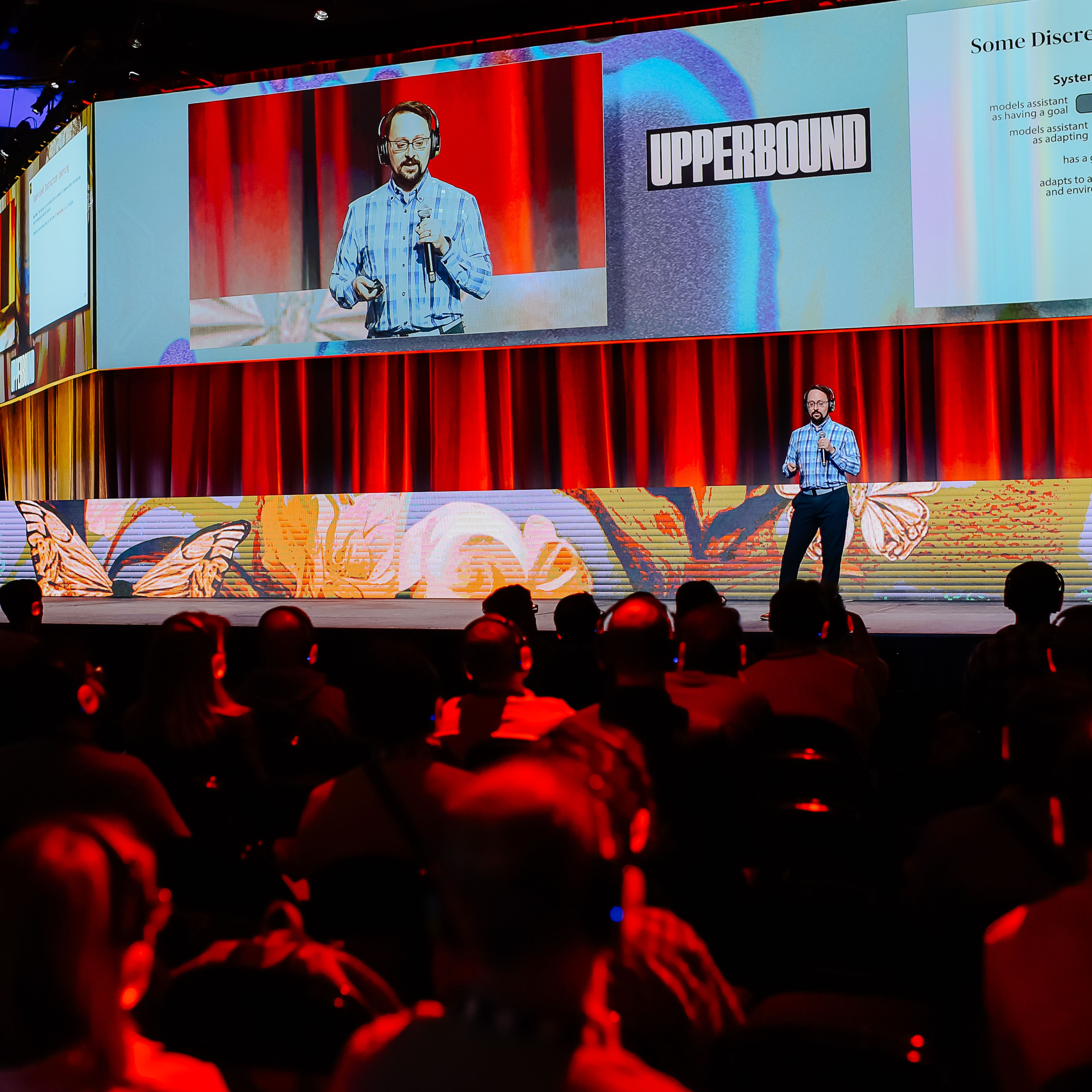
Not Your Average AI Conference
Learn from Leading Minds in AI at Upper Bound
Be one of the thousands of AI professionals, researchers, business leaders, entrepreneurs, investors, and students in Edmonton this spring. Explore new ideas, challenge the status quo, and help shape a positive AI future.