Richard Sutton and Andrew Barto Recognized for Foundational Reinforcement Learning Research
ACM, the Association for Computing Machinery, today named Amii Fellow & Canada AI Chair Richard S. Sutton and Professor Emeritus of computer science at University of Massachusetts Amherst Andrew G. Barto as the recipients of the 2024 ACM A.M. Turing Award for developing the conceptual and algorithmic foundations of reinforcement learning. In a series of papers beginning in the 1980s, Barto and Sutton introduced the main ideas, constructed the mathematical foundations, and developed important algorithms for reinforcement learning—one of the most important approaches for creating intelligent systems.
The ACM A.M. Turing Award, often referred to as the “Nobel Prize in Computing,” carries a $1 million prize with financial support provided by Google, Inc. The award is named for Alan M. Turing, the British mathematician who articulated the mathematical foundations of computing.
Barto and Sutton, jointly and with others, developed many of the basic algorithmic approaches for RL. These include their foremost contribution, temporal difference learning, which made an important advance in solving reward prediction problems, as well as policy-gradient methods and the use of neural networks as a tool to represent learned functions. They also proposed agent designs that combined learning and planning, demonstrating the value of acquiring knowledge of the environment as a basis for planning.
Perhaps equally influential was their textbook, Reinforcement Learning: An Introduction (1998), which is still the standard reference in the field and has been cited over 75,000 times. It allowed thousands of researchers to understand and contribute to this emerging field and continues to inspire much significant research activity in computer science today.
Although Barto and Sutton’s algorithms were developed decades ago, major advances in the practical applications of RL came about in the past fifteen years by merging RL with deep learning algorithms (pioneered by 2018 Turing Awardees Bengio, Hinton, and LeCun). This led to the technique of deep reinforcement learning.
The most prominent example of RL was the victory by the AlphaGo computer program over the best human Go players in 2016 and 2017. Another major achievement recently has been the development of the chatbot ChatGPT. ChatGPT is a large language model (LLM) trained in two phases, the second of which employs a technique called reinforcement learning from human feedback (RLHF), to capture human expectations.
Rich Sutton: Ideas Matter
Rich Sutton has dedicated his career to understanding the mind, what he calls "one of the few great goods in the universe."
Learn more about his unique way of thinking, and the immense impact he's had on the science of AI.
Read now
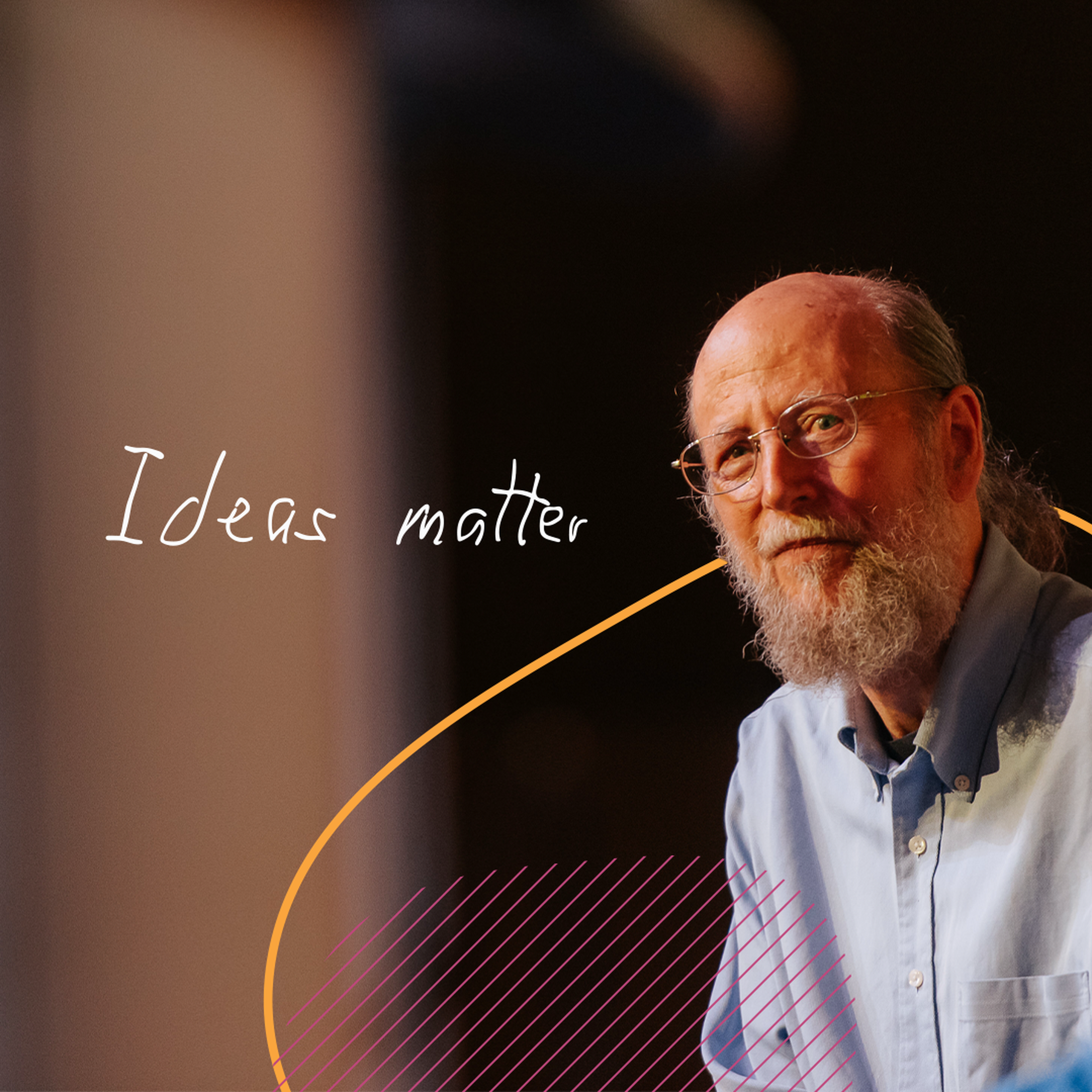
RL has achieved success in many other areas as well. A high-profile research example is robot motor skill learning in the in-hand robotic manipulation and solution of a physical (Rubik’s Cube), which showed it possible to do all the reinforcement learning in simulation yet ultimately be successful in the significantly different real world.
Other areas include network congestion control, chip design, internet advertising, optimization, global supply chain optimization, improving the behavior and reasoning capabilities of chatbots, and even improving algorithms for one of the oldest problems in computer science, matrix multiplication.
“Barto and Sutton’s work demonstrates the immense potential of applying a multidisciplinary approach to longstanding challenges in our field,” explains ACM President Yannis Ioannidis.
“Research areas ranging from cognitive science and psychology to neuroscience inspired the development of reinforcement learning, which has laid the foundations for some of the most important advances in AI and has given us greater insight into how the brain works. Barto and
Sutton’s work is not a stepping stone that we have now moved on from. Reinforcement learning continues to grow and offers great potential for further advances in computing and many other disciplines. It is fitting that we are honoring them with the most prestigious award in our field.”
“In a 1947 lecture, Alan Turing stated ‘What we want is a machine that can learn from experience,’” noted Jeff Dean, Senior Vice President, Google.
“Reinforcement learning, as pioneered by Barto and Sutton, directly answers Turing’s challenge. Their work has been a lynchpin of progress in AI over the last several decades. The tools they developed remain a central pillar of the AI boom and have rendered major advances, attracted legions of young researchers, and driven billions of dollars in investments. RL’s impact will continue well into the future. Google is proud to sponsor the ACM A.M. Turing Award and honor the individuals who have shaped the technologies that improve our lives.”