When Alejandro Coy first heard about Amii’s ML Technician I Course at the AI Meetup in Calgary, the idea excited him. He had been programming in his free time for a few years, which led him to consider a career in data science and machine learning. In the ML Tech certificate, he saw an opportunity to bring his passion into his day job.
Originally from Colombia, Alejandro came to Canada to complete his Master’s degree in Petroleum Engineering at the University of Calgary. When his work as a chemical engineer started to slow down in the summer of 2019, he decided the time was right to take the plunge and apply for the ML Technician I Course.
“Five years ago, I started programming in my free time. I started doing JavaScript and trying to create a webpage -- simple stuff, but fun. It was my hobby,” he explained. “And maybe two or three years ago, I started looking into data science and how I could apply programming to my job. That was my objective: programming in my job all day, not just on the weekends.”
Receiving the acceptance email for the program was a validating moment for Alejandro. The application posed technical questions, and acceptance signalled to him that he was on the correct path. And as an individual whose employment had been negatively impacted by COVID-19 and who belonged to an underrepresented community in STEM (Science, Technology, Engineering & Math), Alejandro’s application was perfectly aligned with the priorities of the Microsoft scholarship.
“I was fortunate to apply for financial support, because I didn’t have much engineering work during the semester...so that was really helpful, too. I am very grateful for it.”
Finding a new perspective
The first few sessions in the program comprised fundamental information to ensure all students were on the same page. After establishing a base of knowledge, they moved into more complex technical subjects and introduced business topics.
"We had a couple of really interesting talks during the course that showed you how you apply that in real life, real projects."
Alejandro Coy
ML Tech I Graduate
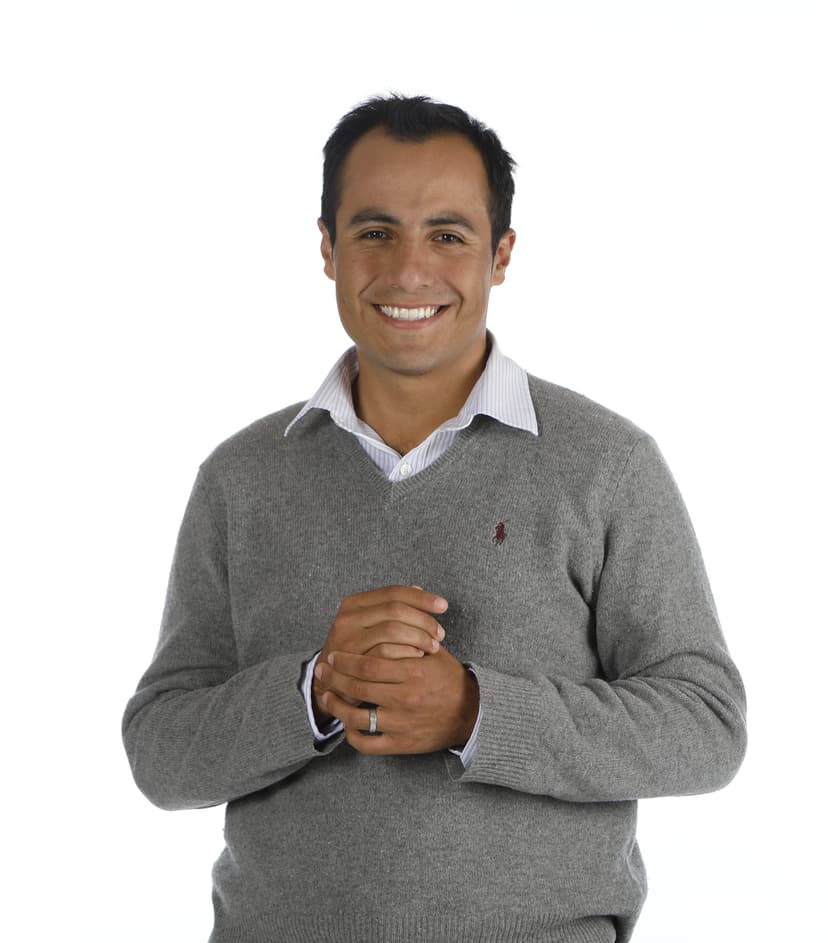
“When we moved to the business module, it was really, really interesting. Online, you can find courses and tutorials teaching you how to do machine learning or data science, but not the application in industry,” he explained. “We had a couple of really interesting talks during the course that showed you how you apply that in real life, real projects. I really enjoyed that. It gave me a different perspective.”
“It's not just the technical skills that you have to know. You need to see the problem in context, how you're going to apply it. Yes, you can get a solution with high accuracy -- but what does that mean?“
As ML Tech I is project-based, students are assigned into groups for the duration of the course; then, over 13 weeks, students complete several programming milestones, all of which contribute to the end-of-course capstone project. These projects mimic the kinds of conditions learners can expect when working on an ML solution in a business environment.
“Since the beginning, we were in one group, and we all have different skills, levels of coding and solving problems. That was my biggest challenge, but I think that it was good because you have to learn to work with people with different skill sets. When you are in the work setting, that is going to happen.”
Putting together the project pieces
As part of the capstone project, each group was required to present their solution to industry and domain experts. This is meant to prepare students for delivering technical presentations to management in a compelling and informative way, explaining how the product works and its benefits.
"We learned so much during the course that when we arrived at the final project, we wanted to include more."
Alejandro Coy
ML Tech I Graduate
“Our business case was to show NASA that we can predict engine failure in a range of 25 cycles. You give me data for one engine, I can tell you if in the next 25 cycles it's going to fail or not. This affects the maintenance schedule and scaling of the use of the engines. If we can narrow that window, it's going to save money in the long term.”
By the time Alejandro’s group had arrived at the capstone project, they found that they had learned so much during the course that they were able to go back to the assignments they had completed earlier, improving and expanding on them.
“I think that the order of the assignments is very logical. We learned so much during the course that when we arrived at the final project, we wanted to include more -- it was a progression. “
Presentation day
Alejandro’s group went above and beyond a standard slideshow, creating a Dash app to work alongside their presentation. Using the app, they were able to display data in an interactive way, allowing them to run scenarios, filter data and change graphs in real-time.
“It really felt like we were presenting the project to managers. The business component was the most important stuff for me because a big part of the final project is how you present it, how you explain the objectives and how you convince the managers to go for the project.”
And in the end, how did they do? According to Educator Scientist at Amii and ML Tech I Course teacher, Blanca Miller:
"This group’s project stands out as one of the top projects we’ve seen so far in this course."
Blanca Miller
ML Tech I Course Teacher
“This group’s project stands out as one of the top projects we’ve seen so far in this course. The group put in a lot of work to ensure that the evaluations of their machine learning models aligned with the strategic goals of their organization, NASA, and they also developed a compelling business case with dynamic visualizations, which made the material accessible for both scientists and business experts -- this was largely due to Alejandro’s efforts.“
“After the presentation, we talked about how it went, and we were very happy,” Alejandro shared. “It was a lot of work to get to that point, and it was a relief that everything actually worked. We had a really good business case, and we showed people how impactful it could be. We were very proud of what we did.”
Conclusion
Alejandro now works as a Senior Data Specialist, Data Efficiencies at Alberta Investment Management Corporation (AIMCo). His hobby, which he once pursued on evenings and weekends, has become his career.
"In my job interview, I presented the capstone project and they were very impressed."
Alejandro Coy
ML Tech I Graduate
“It has been two months since I started working at AIMCo and I couldn’t be happier. Amii training was a huge factor and I learned about the job thanks to networking in the course. In my job interview, I presented the capstone project and they were very impressed."
He maintains that the most valuable thing he learned from the course is that ML is applicable across industries.
“It's not just tech stuff,” says Alejandro. “It has a wide variety of applications, and it's not that difficult to implement. Most situations in real life, you don't need that complexity. You can apply machine learning to many problems without having a complex model -- just have to start with a base model, and you can figure out if you are on the right path or not.”
Authors
Britt Ayotte