Advances in representation learning
Martha White’s primary research goal is to develop techniques for adaptive autonomous agents learning on streams of data. Over the past years, she has focused on principled optimization approaches for representation learning, particularly looking at sparse representations and recurrent architectures for partially observable domains. Martha has also been working on off-policy reinforcement learning, which enables learning about many different policies in parallel from a single stream of interaction with the environment. Overall, she seeks to make advances in representation learning for reinforcement learning, which she believes is one of the biggest scientific hurdles for AI and autonomous agents.
Martha is a Fellow and Canada CIFAR AI Chair at Amii and an Associate Professor in the Department of Computing Science at the University of Alberta.
She has delivered invited talks at the International Conference on Learning Representations (ICLR), the International Conference on Machine Learning (ICML), and Advances in Neural Information Processing Systems (NeurIPS). She has also acted as area chair at these conferences as well as at the AAAI Conference on Artificial Intelligence.
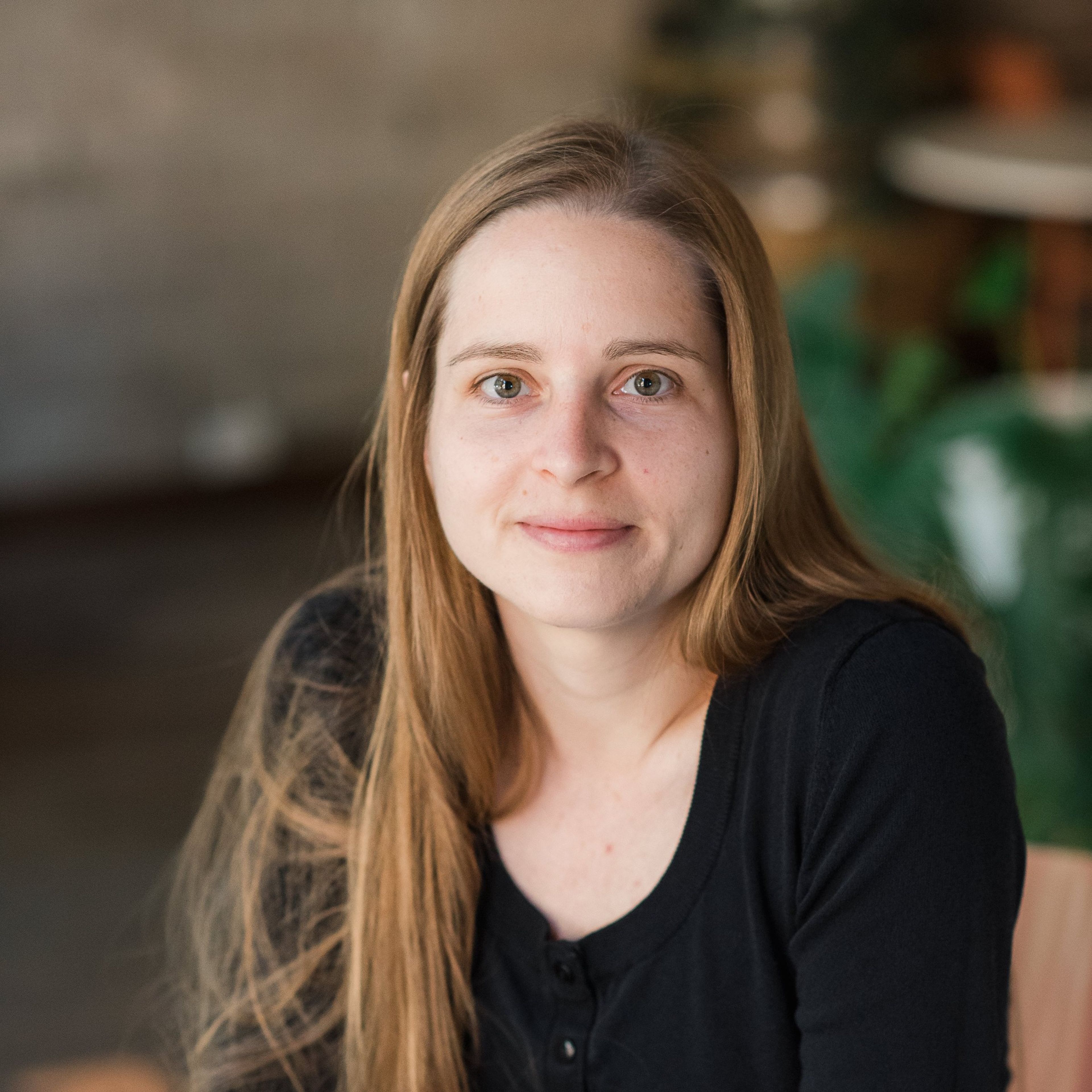
Martha also devotes some of her time to mentoring and promoting diversity in computing science. Since obtaining her Ph.D. in Computing Science from the University of Alberta in 2014, Martha has supervised more than 30 early-career researchers at the M.Sc. and Ph.D. levels as well as Research Associates and Post-Doctoral Fellows and has authored more than 40 papers in top journals and conferences.
Areas of
Expertise
Reinforcement learning
Representation learning
Time series prediction
Off-policy learning